„We see the forest, not just the trees“

Limited, but incredibly powerful
Mr. Mayer-Schönberger, Mr. de Véricourt, it is often claimed that human decisions are inferior to those of machines – due to personal biases, for example. You think that's wrong. Why?
Francis de Véricourt: Humans do indeed have cognitive limits. For more than three decades, research in psychology and decision science has revealed the many ways in which we make bad decisions. Psychologist and Nobel laureate Daniel Kahnemann described this with the concept of "Thinking, Fast and Slow." But human decision-making can also be incredibly powerful. By focusing solely on the downsides of human decision-making, we overlook our strength, a unique ability that neither animals nor machines have: that of framing. It is time to focus on this cognitive ability because it can help us solve humanity's most pressing problems.
By focusing solely on the downsides of human decision-making, we overlook our strength, a unique ability that neither animals nor machines have: that of framing.
What do you mean by framing?
Viktor Mayer-Schönberger: Frames are mental models, the way we imagine the world in our heads. These models have three components. First, we use them to develop causal assumptions about how the world works. This, in turn, allows us to dream up alternative realities and develop a set of decision options from them. Third, in doing so, we narrow down our dreams by focusing on the most important options and ignoring unimportant ones by setting constraints.
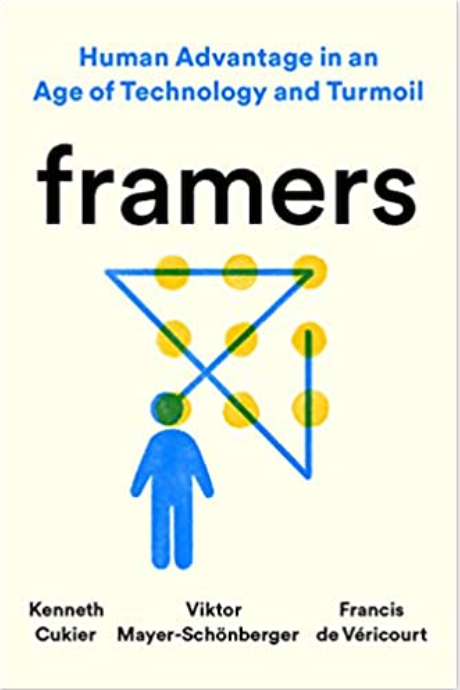
An example?
Francis de Véricourt: The founders of Biontech foresaw as early as January 2020, when they were a small start-up, that there would be a massive pandemic, well before many others, such as the pharmaceutical company Pfizer. At the time, however, there wasn't much data available – the number of cases was quite small, concentrated in Wuhan, China, and the disease was largely unknown. So how was it possible for Biontech to anticipate this huge pandemic? How could they look so far ahead from the little information they had and still be right? Biontech could because they applied the right frame: an abstract representation that captures the way the virus spreads - via network effects - in populations. In this way, they could envision the consequences of a global pandemic, something we've never seen on the scale. And they were so convinced by what they saw, that they decided to devote all their resources to develop a vaccine. It was only a few weeks after the virus reached the rest of the world before Pfizer realized that Biontech was right and joined them.
AI can predict the future by extrapolating from the past. If the future is like the past, that works well. But when the future is fundamentally different from the past, AI fails.
But when it comes to developing new products, especially in healthcare, there are always stories about how AI is making a difference. Is this all nonsense?
Francis de Véricourt: Machine learning and AI can help people when there are large amounts of data to process. For that, the data you train an algorithm on has to be representative. Algorithms can't infer much beyond the data they're trained on - they're sort of locked into that data. If there is very limited data, as there was at the beginning of the Corona pandemic, AI can't help. In contrast, humans can use their imagination to come to better decisions that go beyond the available data. This is possible because we are able to properly represent and frame the situations we find ourselves in.
How was it possible for Biontech to anticipate this huge pandemic? They could because they applied the right frame: an abstract representation that captures the way the virus spreads - via network effects - in populations.
Viktor Mayer-Schönberger: AI can predict the future by extrapolating from the past. If the future is like the past, that works well. But when the future is fundamentally different from the past, AI fails. AI is phenomenal at uncovering patterns hidden in data, but we humans make sense of those patterns - we see the forest, not just the trees. AI is great to drive efficiencies in a stable environment – optimizing what already exists. But today we're in a time where we're facing big challenges in business and society, whether it's the pandemic, digitalization, or global warming. AI can do little about that.
Artificial Intelligence can help HR – but not always
HR departments are discussing the use of AI in recruiting. Human prejudices or our tendency to prefer candidates who are similar to us can affect the selection process. Are machines the better recruiters?
Viktor Mayer-Schönberger: It depends. If your HR problem is categorizing candidates in known buckets, AI is better. Because AI recognizes patterns and can categorize people. But when job profiles change rapidly and employees are expected to fulfill new roles on an ongoing basis, AI is of little help. So whether AI is an effective tool depends on the needs of companies in the future, i.e. which jobs it will create and how flexible employees' roles should be.
Francis de Véricourt: Machines can provide information about employees' personal networks or even their past performance - as a basis for decision-making. But hiring decisions are often about predicting how someone will perform over several years in an environment that is unknown today, and sometimes for positions that didn't exist before. It is impossible to find fully representative data to train a machine to do this. In addition, many HR decisions require explanation - for example, why certain employees get bonuses or promotions and others don't. But machines don't provide a rationale for their decisions.
You harm people when you try to get them to think outside the box. People think in frames, which means they constrain their thinking. The magic is in the box.
When it comes to activating the imagination in management circles, people often say that you have to "think outside the box". You think that's wrong. Why?
Francis de Véricourt: 30 years of research on the subject show that you harm people when you try to get them to think outside the box. They are then less creative, less innovative, and make worse decisions. People think in frames, which means they constrain their thinking. If you ask someone to think without constraints, quite wild ideas emerge - but they no longer relate to reality or solve the problem. The magic is in the box.
Think inside the box!
That means, even if we use new frames, we think in a box? A different box, but still a box?
Viktor Mayer-Schönberger: Yes, exactly. And the most important thing: Even if thinking in a different box sounds tempting, you should be careful about changing the box. Because most of the time we fail when trying it. Therefore, it is better to make full use of the possibilities of the mental model we are in and familiar with and play with the model's constraints. Most of our mental models are far more flexible and adaptable than we think. By exercising the constraints to push the limits of our model, we produce better decision options.
Most of our mental models are far more flexible and adaptable than we think. By exercising the constraints to push the limits of our model, we produce better decision options.
Do you have an example of this?
Viktor Mayer-Schönberger: In 1978, the mountaineers Reinhold Messner and Peter Habeler climbed Mount Everest for the first time without supplemental oxygen. That was a sensation. Because a persistent lack of oxygen at high altitudes can lead to death when exposed to it for too long. Therefore, before that, in expedition style, oxygen and provisions were brought higher and higher. But Messner and Habeler realized that one constraint could be adjusted in the mental model of high-altitude mountaineering: speed. If you run up and down the mountain really fast, the lack of oxygen is short-lived and inconsequential. It worked and started a revolution, it opened new possibilities. But it wasn't a new frame. They did not beam themselves to the summit, they were still mountaineers.
And AI is really not supposed to be able to do that, to play with constraints in this way?
Viktor Mayer-Schönberger: Machines can permutate constraints, but they lack the ability to dream purposefully and thus reduce countless decision options to essential ones. Take AlphaZero from Google subsidiary DeepMind, for example. This phenomenal AI system learned to play chess by playing against itself, only to win against top human players. It caused a storm in the chess community because AlphaZero played chess very differently than human players and still won. And a lot of people thought, wow, that's really creative. But AlphaZero has no understanding whatsoever of what it's doing. It can't generalize. Machines just sample the elements systematically. AI recognizes patterns, but when faced with another situation, it has to keep starting over. Maybe it will find a good solution by chance at some point, but maybe 1,000 years too late.
Machines can permutate constraints, but they lack the ability to dream purposefully and thus reduce countless decision options to essential ones.
Francis de Véricourt: Machines score points for computing speed. They are able to sift through huge amounts of data. But for this to lead to good results, humans have to find the relevant data, which depends on how you formulate the problem in the first place. That was the case, for example, with the discovery of the new antibiotic Halicin at MIT. Usually, in drug development, researchers try to find substances that have similar molecular properties to existing antibiotics. But the MIT researchers viewed the challenge as an information problem that was less about the structure and more about the function of the substance: does it kill bacteria? They then carefully selected databases of chemical compounds for their AI system to examine. What was hailed as a triumph of AI was a success of human framing.
Take the new antibiotic Halicin discovered at MIT. What was hailed as a triumph of AI was a success of human framing.
But people also find it very difficult to change frames...
Viktor Mayer-Schönberger: Yes, that's true. The car industry is very important in Germany and has been successful worldwide. But why is the leading company for electric vehicles an American start-up? It's not because of technology, but because of the power of the mental model. Focused on what they were good at, Daimler and BMW saw cars as internal combustion engine vehicles. That was their frame. Tesla, on the other hand, saw cars as something that needed a motor and a power source. So your own success can prevent you from leaving familiar paths to think new things.
We can change frames
You say that companies are more successful and adaptable when they use different frames. So, from your point of view, does diversity make you more innovative?
Francis de Véricourt: With all due caution when it comes to changing frames, sometimes it is simply necessary in order to adapt to new situations. The more frames an organization can use, the better equipped it is to do so. In the wake of the U.S. subprime crisis in 2007, investment bank Merrill Lynch was forced to take balance sheet write-downs in the billions of dollars. CEO Stanley O'Neal was caught completely off guard by this and had to take his hat a short time later. The reason: He didn't include people on his team who had a different view of how the market works. So there was a strong tendency to kill the diversity of frames in the company. When everyone follows the same business model or has the same views, it may produce a nice feeling. But it is also dangerous. Conflict aversion and the desire for a "shared frame" is so great that diversity is too often destroyed in the name of efficiency.
There is no such thing as a bad frame, with one exception: frames that deny other frames the right to exist, such as the frames of Nazism or Communism.
Viktor Mayer-Schönberger: In the 1980s, people in Germany talked about Japan being the future economic superpower. Japanese companies were very well known for their relentless pursuit of efficiency. And this works wonderfully as long as no one has a fundamentally different idea of what is needed in the market. But becoming more efficient at making analog cameras is a futile endeavor when everyone has a smartphone with a digital one. So Japan has been going through an economic slump for 25 years. Because there is a cognitive monoculture, not only in the big companies but in the Japanese economy as a whole.
Should one accept all mental models in principle? Or in your opinion are there also bad frames that do not work in principle?
Viktor Mayer-Schönberger: There is no such thing as a bad frame, with one exception: frames that deny other frames the right to exist, such as the frames of Nazism or Communism. One cannot live in a world with only one frame – we would die in efficiency. It is important to engage with other frames, even those we reject - if only to understand how others see the world. Whether a frame is a “good” one depends crucially on the situation: When I measure my living room, I can pretend there is no curvature of the earth. On the other hand, that would be completely wrong if we were sending rockets into space.
Francis de Véricourt: We often apply mental models that don't fit the situation or the context - that's what we call "misframing". The context is important. A mental model is like a screwdriver: it's a useful tool, but a bad idea to hammer in a nail with it.
To what extent can you learn framing or improve framing skills?
Francis de Véricourt: Framing is like a muscle that you should train. First, you can ask yourself under what conditions you are currently working. Often, out-of-tune constraints are unconsciously present. Then you should try to ask what-if questions: What if I loosened this constraint? What if I omitted another one altogether? In this way, one can find new alternatives and stimulate counterfactual thinking. So you can start changing the constraint within your own framework to see where that takes you. The next step is to try to expand one's repertoire of frames. That means taking our curiosity seriously. Personally, I'm reading something about Mayan history right now. Not that I have a particularly strong interest in that civilization, and I don't want to become an expert in it, but I am curious to learn how people so far away from me in time and space solved their problems. Maybe someday I'll get into a situation where these frames work well.
The context is important. A mental model is like a screwdriver: it's a useful tool, but a bad idea to hammer in a nail with it.
Viktor Mayer-Schönberger: It's not only nonfiction books that help, but also good novels. When we read, we can imagine an alternative reality and imagine what happens in the next chapter. That also applies to going to the theater or playing video games.
Can companies specifically support framing with their continuing education offerings?
Francis de Véricourt: Business schools traditionally work with case studies. Students are asked to find a solution to a specific problem. To help them, they are given frames - from finance or marketing, for example. As instructors, we present different points of view and the students can consider which of them fits best. So a good business school offers much more than just one methodology or technique: It offers the opportunity to learn about and apply as many different frames as possible.
Business schools are primarily driven by the frame of profit maximization and economic key figures. How does that fit in with new requirements, for example, that companies should operate more sustainably?
Francis de Véricourt: Of course, paradoxes arise when it comes to sustainability. You want to earn money and at the same time treat the environment with care. But these apparent contradictions can also lead to innovative solutions.
Viktor Mayer-Schönberger: You should ask yourself: What are mutable constraints and what are hard, immutable ones? When people think about heating and the enormous environmental impact, for example, one frame focuses on the production of energy. People for instance want to use heat pumps to generate energy. But you can look at the problem from another angle. An architecture firm has determined that it is possible to do without central heating altogether by using human heat and the excess heat from electrical appliances. Construction and heating costs are 30 percent lower. An example of purposeful dreaming: energy does not always have to be generated by a dedicated machine, it can be a byproduct.
Francis de Véricourt: In 2009, I did a case study for a large European logistics company. Being a transport company, its carbon footprint was obviously a problem. So the company implemented what they called a green program to find ways to reduce those emissions. It turned out that this initiative had a profound impact on the employees' mindset. It caused them to think less in silos and consider potential synergies within the company, as well as with their suppliers. It helped the company frame some of its business problems differently. As a result, the company found innovative solutions that improved its bottom line while reducing its carbon emissions. Of course, such a win-win situation won't always happen. But framing opens up new solution options.
Framing is a power we all possess. If we take it seriously and apply the necessary discipline, we can all become better framers.
To what extent is there a danger that framing as the most important source of future employability is only open to an elite group at business schools and not to those who are particularly affected by job losses due to AI?
Francis de Véricourt: Framing is a power we all possess. If we take it seriously and apply the necessary discipline, we can all become better framers. And any educational program can take up this approach. It doesn't require a huge investment. It's really about having the right mindset.
This interview was first published in German in personalmagazin 4/22, Germany's leading HR magazine.